Streamlining Data Operations with a Metadata-Driven Data Lakehouse on Azure
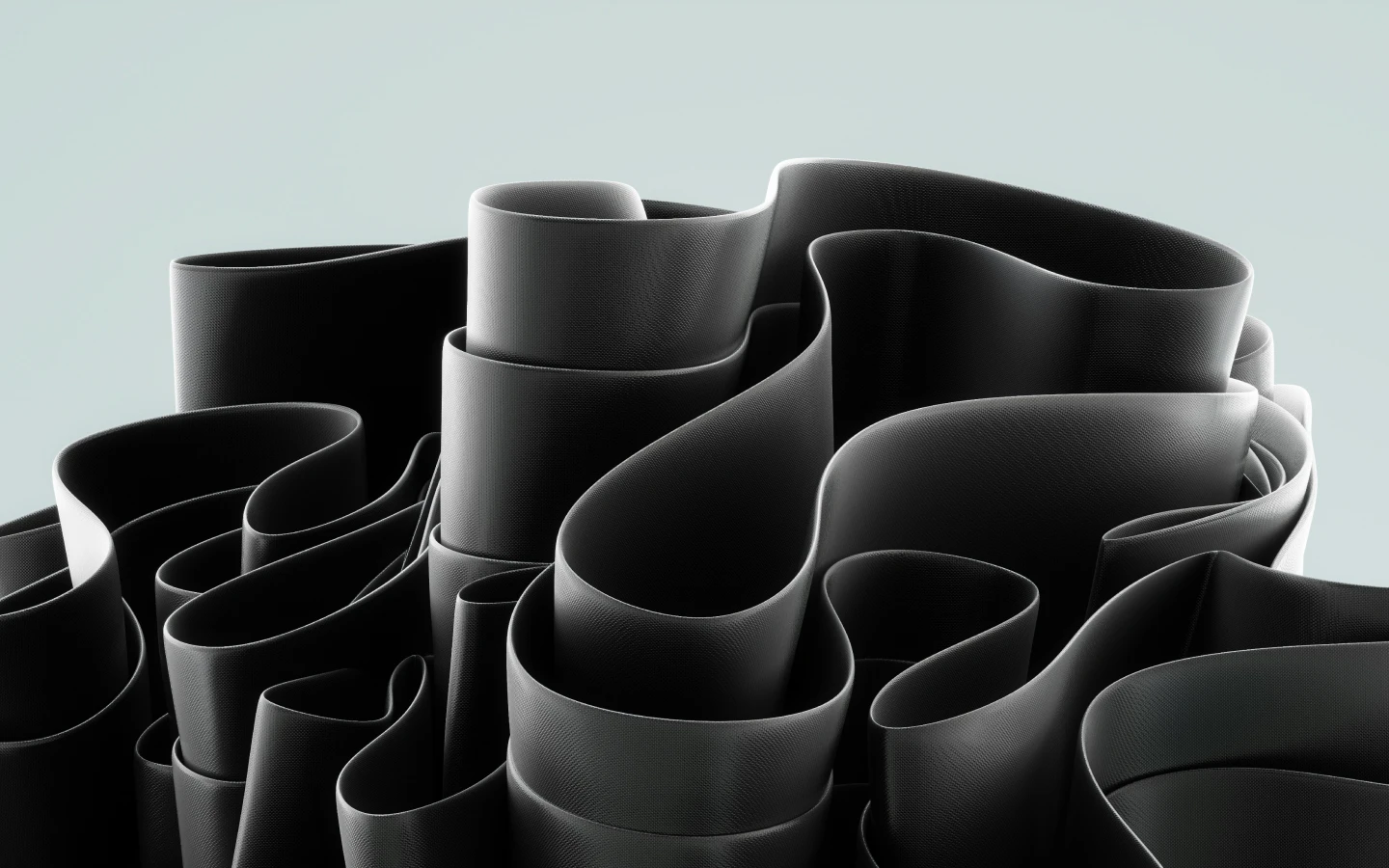
Challenge
A Fortune 500 FMCG company struggled with their existing Azure Databricks data lake solution, which was plagued by complexity, duplicated datasets, and a lack of structure. They required a streamlined solution to simplify data operations, enhance data quality, and improve data discoverability, all while optimizing costs.


Our approach
Our team launched a transformative project to migrate the client’s Azure Databricks data lake to a metadata-driven data lakehouse using the medallion architecture. By leveraging Databricks, Python, Azure, and Spark, we implemented a scalable and organized solution that enforced the medallion structure and improved data quality without disrupting user workflows.
Key components of the solution included:
- A metadata-driven framework for data pipeline automation, incorporating features like automatic data extraction, archiving, and incremental load support.
- Seamless medallion structure enforcement that maintained user-friendly flexibility.
- Integration of Great Expectations for automated data quality checks and validation.
The outcome
The migration to a metadata-driven data lakehouse delivered substantial improvements in data discoverability and usability. The medallion architecture provided a clear structure, enabling citizen developers to directly engage with datasets, fostering self-service analytics and innovation.
Additionally, automation features, including data extraction, archiving, and incremental loads, significantly reduced pipeline costs and enhanced operational efficiency. Integrating Great Expectations ensured data integrity and reliability, meeting high-quality standards.


Business Impact
The project revolutionized the client's data operations by streamlining processes and enhancing data management. This scalable and efficient solution empowered the client to unlock the full potential of their data assets, driving self-service analytics, operational cost savings, and faster decision-making in the competitive FMCG industry.
"Lorem ipsum dolor sit amet, consectetur adipiscing elit. Suspendisse varius enim in eros elementum tristique. Duis cursus, mi quis viverra ornare, eros dolor interdum nulla, ut commodo diam libero vitae erat."
Browse more projects
Let’s talk and work together
We’ll get back to you within 4 hours on working days (Mon – Fri, 9am – 5pm CET).

Service Delivery Partner